How AI is Transforming Science and Medicine
- Juan Manuel Ortiz de Zarate
- 10 minutes ago
- 11 min read
Artificial Intelligence (AI) is no longer a futuristic promise in the realms of science and medicine—it is the present. In 2025, breakthroughs in clinical diagnostics, protein engineering, and drug discovery reflect how AI is reshaping research and practice. Stanford University’s 2025 AI Index Report [1], particularly Chapter 5—produced in partnership with RAISE Health—paints a detailed picture of this transformation. This article highlights key advancements from the report and explores how AI is not only accelerating scientific discovery but also enhancing patient care.
AI as a Virtual Scientist: The Rise of Autonomous Labs
Artificial intelligence is no longer confined to acting as a tool for human researchers—it is now stepping into the role of scientist itself. One of the most groundbreaking developments in 2024 was the emergence of virtual AI laboratories, systems composed entirely of AI agents that operate collaboratively to conduct research autonomously. These digital labs mark a radical shift in the scientific method, where human intuition and manual experimentation are increasingly supplemented—or even replaced—by coordinated AI-driven discovery.
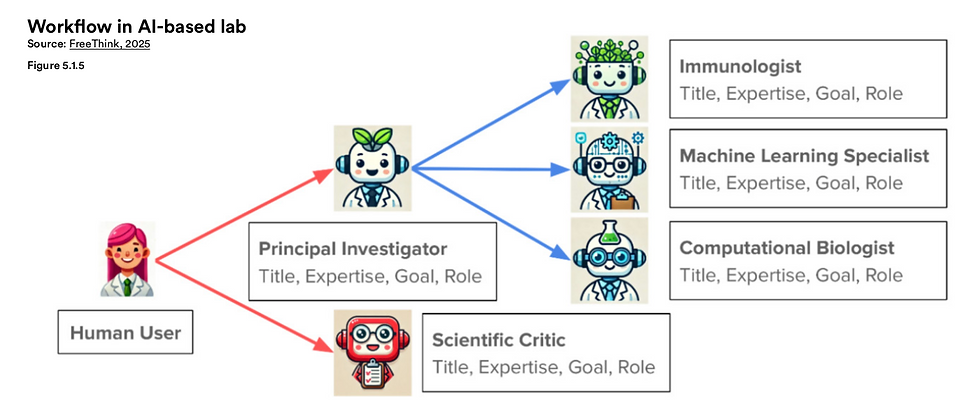
A landmark study from Stanford University showcased this transformative idea in action. Researchers designed a virtual lab composed of large language models (LLMs) that specialized in different scientific domains. These agents functioned under the direction of an AI-based "Principal Investigator" and were tasked with designing nanobodies—antibody fragments capable of binding to the SARS-CoV-2 virus. Working entirely in silico, this AI laboratory produced 92 unique nanobodies, and impressively, over 90% demonstrated effective binding in experimental validation. The implications are profound: a team of autonomous agents, with minimal human intervention, was able to generate real-world scientific outcomes with biomedical relevance.
The virtual lab was structured to resemble the dynamics of a multidisciplinary research team. Alongside the PI, there were agents specializing in immunology, computational biology, and machine learning. Each agent contributed domain-specific insights, and a separate AI model acted as a scientific critic—assessing ideas, challenging assumptions, and refining the research direction. This setup mirrors the collaborative nature of human labs, but with two distinct advantages: speed and scale. AI agents never tire, can instantly ingest entire libraries of scientific literature, and can iterate through hypotheses at rates unattainable by human teams.
This is part of a broader trend often referred to as agentic AI—AI systems that do not simply execute predefined tasks, but reason through problems, make decisions, and interact with tools and datasets to achieve goals. One related example is Aviary, a framework for training LLM-based agents to solve complex scientific tasks. These include DNA manipulation for molecular cloning, protein stability optimization, and the answering of research questions by parsing scientific papers. In benchmarks, agentic models using Aviary environments consistently outperformed non-agentic baselines, demonstrating that the structure and interactivity of these systems significantly enhance their problem-solving ability.
This evolution holds profound implications for the future of research. Virtual AI labs could democratize access to cutting-edge science, allowing smaller institutions or underfunded labs to leverage powerful models trained on the world’s knowledge. Moreover, such systems might one day be tasked with exploring hypotheses deemed too risky, slow, or expensive for traditional labs, accelerating discovery in areas like rare disease research, synthetic biology, or climate modeling.
Yet the rise of autonomous labs also raises new questions: How do we validate discoveries generated entirely by machines? Who owns the intellectual property? And how do we ensure accountability when AI designs a failed experiment—or a breakthrough?
These are the early days of what could be the most significant paradigm shift in science since the invention of the microscope. The scientist of the future may not wear a lab coat—but rather reside in the cloud.
Mapping the Human Brain and Managing Chronic Illness
Among the most awe-inspiring scientific milestones of 2024 is a feat that once seemed like science fiction: mapping a piece of the human brain at synaptic resolution. Spearheaded by Google’s Connectomics project [4], this initiative successfully reconstructed a one-cubic-millimeter section of the human cerebral cortex—equivalent to a grain of sand—at unprecedented detail. While tiny in size, this sliver of tissue revealed a staggering 150 million synapses, over 57,000 cells, and intricate interactions among neurons, glial cells, and blood vessels.
The data acquisition process involved over 5,000 ultra-thin slices, each just 30 nanometers thick, captured using a multibeam scanning electron microscope. This raw imaging data was then processed using advanced machine learning tools, including flood-filling networks for 3D neuron reconstruction and SegCLR for identifying different cell types. To manage and explore such a vast dataset, Google deployed TensorStore and visualization platforms like Neuroglancer and CAVE, offering open access to researchers around the world.

This monumental project represents more than a technological achievement—it offers a blueprint for decoding the human mind. By examining the detailed architecture of excitatory and inhibitory connections, researchers hope to uncover the biological underpinnings of cognition, memory, and neuropsychiatric disorders. In the long term, this work could drive advances in treating epilepsy, Alzheimer’s, and brain injuries, where understanding synaptic patterns is key to intervention.
While the brain mapping project illuminates the most complex organ in the body, other AI models are targeting some of its most chronic challenges. One such innovation is GluFormer[5], a foundation model developed collaboratively by Nvidia Tel Aviv and the Weizmann Institute. Trained on more than 10 million glucose measurements from nearly 11,000 individuals—most of whom do not have diabetes—GluFormer can forecast a person’s glycemic health up to four years in advance.
Its ability to predict the onset of type 2 diabetes, worsening glycemic control, or even cardiovascular-related mortality represents a major leap forward in preventive medicine. In a 12-year cohort study, GluFormer accurately flagged 66% of new-onset diabetes cases and 69% of cardiovascular deaths within their highest-risk quartiles. Even more impressively, the model generalized well across 19 external cohorts from five countries, showcasing its robustness and fairness across populations.
GluFormer is part of a larger shift in chronic disease management—from reactive care to proactive risk prediction. Instead of waiting for symptoms to emerge, physicians armed with AI tools can intervene earlier, personalize treatments, and allocate resources more efficiently. These kinds of predictive models promise to reduce hospitalizations, cut healthcare costs, and extend the healthy lifespan of at-risk populations.
In short, from reconstructing the brain’s neural web to forecasting chronic illness years before symptoms appear, AI is moving medicine into a new era—one defined not just by smarter tools, but by a deeper understanding of biology and better anticipation of disease.
AI in Clinical Knowledge: Outscoring the Experts
If there is one area where the power of large language models (LLMs) is being put to the ultimate test, it is in clinical knowledge and reasoning. Unlike pattern recognition in imaging or structured predictions in genomics, clinical decision-making demands nuanced understanding, contextual reasoning, and the ability to weigh risk, ambiguity, and ethics. In 2025, AI systems are proving they can not only meet these challenges—but often exceed human expertise.
A major highlight of the 2025 AI Index Report is the performance of OpenAI’s o1 model on the MedQA benchmark [6], a dataset derived from professional medical board exams. Featuring over 60,000 questions that challenge physicians across specialties, MedQA is considered one of the most rigorous tests of clinical knowledge in AI. The o1 model achieved a remarkable 96.0% accuracy, outperforming all previous models by a wide margin and improving by 5.8 percentage points over the 2023 state-of-the-art.
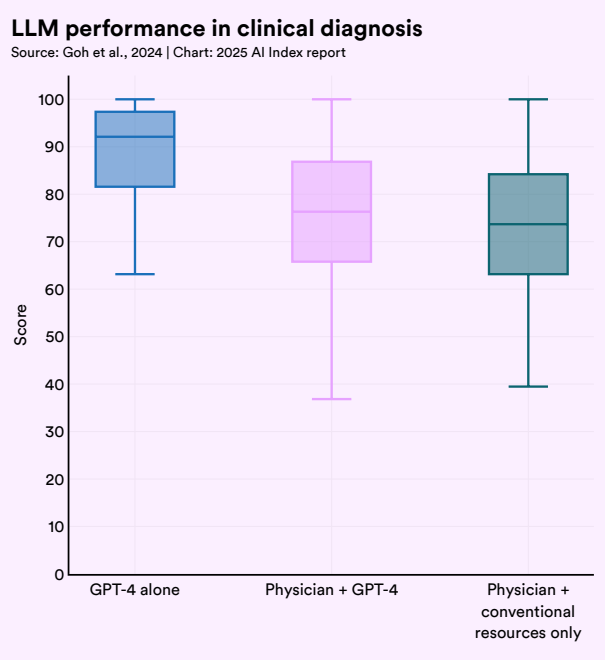
What makes this achievement more striking is that o1 reached this score without additional fine-tuning. Instead, it leverages advanced runtime reasoning—akin to chain-of-thought thinking—which allows the model to simulate clinical reasoning processes in real time. This method mirrors the reflective thinking physicians engage in when weighing symptoms, histories, and comorbidities to arrive at a diagnosis or treatment plan.
To test performance beyond benchmarks, researchers conducted comparative studies involving physicians and AI systems tackling real-world clinical scenarios. In one single-blind randomized trial, 50 licensed U.S. physicians were asked to solve complex diagnostic vignettes, either using conventional resources, GPT-4, or a combination of both. The results were illuminating: GPT-4 alone achieved 92% accuracy, outperforming both doctors with traditional tools (74%) and even doctors assisted by GPT-4 (76%).
However, this doesn’t mean human-AI collaboration is obsolete. Another randomized trial focused on clinical management tasks—decisions involving treatment plans, balancing patient preferences, and evaluating risk. Here, physicians assisted by GPT-4 outperformed their unassisted peers by 6.5 points, although they spent slightly more time per case. Interestingly, GPT-4 on its own performed about the same as the assisted physicians, suggesting that near-autonomous decision support may be viable in certain scenarios, particularly in highly protocolized or well-understood cases.
These findings highlight a subtle but essential truth: excellent standalone model performance does not guarantee effective collaboration. Giving doctors access to powerful AI tools doesn’t always improve outcomes unless workflows, interfaces, and training are carefully designed to integrate the AI in intuitive, trustworthy ways. Bridging this gap between machine excellence and human usability remains a core challenge for the years ahead.
Meanwhile, the sheer pace of innovation is staggering. In 2024 alone, over 1,200 papers on large language models in medicine were published, reflecting a surge in interest and investment in clinical AI. This growing body of research emphasizes not just accuracy, but also cost-efficiency, safety, and ethical deployment—critical factors as LLMs move from academic labs to hospital floors.
As AI systems continue to outperform experts on certain tasks, the future of medicine is not about replacing doctors, but augmenting them with tools that think, reflect, and adapt. It’s not a contest between human and machine—it’s the dawn of a powerful partnership.
Intelligent Infrastructure for Biomedicine: Molecules, Images, and Workflows
Artificial intelligence is rapidly building an intelligent infrastructure that spans the molecular, visual, and procedural layers of modern medicine. From modeling the behavior of proteins to interpreting complex medical imagery and reducing administrative burdens, AI is becoming a multi-modal, multi-purpose engine driving healthcare innovation from the bench to the bedside.
At the molecular level, protein science has been revolutionized by next-generation models. AlphaFold 3 [2], from Google and Isomorphic Labs, advances beyond structural prediction to simulate interactions between proteins and other biomolecules, such as DNA, RNA, and small-molecule ligands. This capability, which has proven more accurate than traditional docking methods—achieving over 93% prediction accuracy in some cases—marks a new era in drug discovery and biochemical research. AlphaFold 3 is especially impactful in modeling how therapeutics bind to their targets, accelerating the pace of preclinical development.
Simultaneously, Evolutionary Scale Modeling v3 (ESM3) has redefined synthetic biology by simulating evolutionary processes to generate novel proteins. The model’s most famous creation to date—esmGFP, an artificial fluorescent protein—represents a leap that nature may have taken hundreds of millions of years to accomplish. With its 98 billion parameters and open-source accessibility, ESM3 has democratized access to frontier protein engineering technology, inviting broader collaboration across academic and industrial domains.
These molecular breakthroughs are complemented by DeepMind’s AlphaProteo [3], a model capable of generating high-affinity protein binders for therapeutic targets. The tool has successfully designed binders for molecules linked to cancer and diabetes, some of which are up to 300 times more effective than current drugs. This kind of AI-guided design could lead to faster, cheaper, and more precise treatment options for a wide range of diseases.
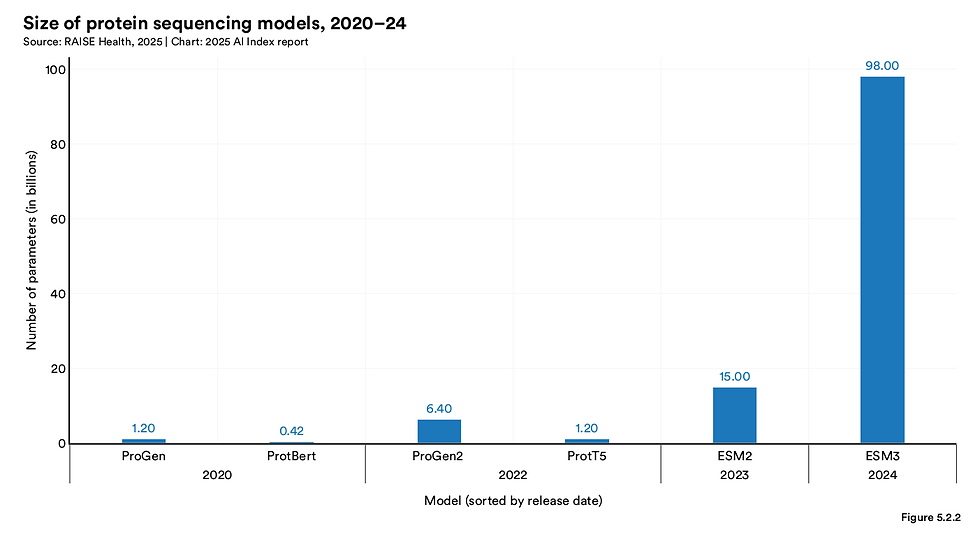
In parallel with molecular advances, medical imaging is undergoing a profound transformation through foundation models like Med-Gemini [7], ChexAgent[8], and EchoCLIP. These vision-language models (LVLMs) integrate radiological images with clinical notes to automate tasks such as diagnosis, segmentation, and report generation. They bring unprecedented accuracy and speed to radiology, cardiology, and pathology, acting as intelligent assistants that contextualize visual data with patient history.
Microscopy—a cornerstone of biological research—is also benefitting from AI. The number of foundation models for light, electron, and fluorescence microscopy doubled in 2024. These models are helping researchers analyze tissues at atomic and subcellular levels, revealing patterns that were previously invisible. However, challenges persist: imaging datasets remain geographically narrow, often concentrated in states like California and New York, and 3D imaging datasets are still scarce. Overcoming these limitations is essential to ensure fairness, robustness, and clinical generalizability.
Beyond molecules and images, AI is streamlining the human side of medicine. One of the most transformative applications is the rise of ambient AI scribes, designed to alleviate the burden of clinical documentation. These systems, powered by large language models, listen to physician-patient conversations and automatically generate notes for the electronic health record (EHR). A Stanford study found that physicians using a fully automated scribe saved an average of 20 minutes per day and reported 35% lower levels of burnout. Adoption is already widespread in institutions like Kaiser Permanente and Intermountain Health.
Investments in scribe technology reached nearly $300 million in 2024, underscoring the growing recognition of AI’s role not just in high-stakes diagnostics, but in everyday clinical efficiency. These systems are now expanding into adjacent areas such as billing, coding, and even real-time clinical decision support, signaling a future where administrative overhead no longer impedes quality care.
Together, these developments show that AI is not limited to a single breakthrough or specialty—it is weaving itself into the core infrastructure of biomedicine, offering new ways to observe, understand, and act across the full continuum of health.
Scaling Responsibly: Regulation, Ethics, and Equity in Medical AI
As AI becomes embedded in healthcare, its success depends not only on performance, but on regulation, ethics, and equity. The surge in FDA-approved AI medical devices—from just six in 2015 to 223 by 2023—signals growing institutional trust. Real-world implementations, like Stanford Health Care’s PAD screening and documentation tools, show that structured deployment frameworks can deliver measurable clinical and financial value.
But with rapid expansion comes responsibility. Medical AI ethics publications have quadrupled since 2020, reflecting concerns about bias, transparency, and safe integration. One emerging solution is synthetic data, which enables research and model development without compromising patient privacy. Tools like ADSGAN and PATEGAN replicate real-world data distributions and are already being used for risk modeling and drug development, accelerating discovery while preserving anonymity.
Equity also demands broader context. By incorporating social determinants of health (SDoH)—such as housing, income, and education—into clinical models [9], AI can move beyond biology to better reflect patients' lived realities. Fine-tuned models like Flan-T5 XL outperform general-purpose LLMs in identifying SDoH from clinical notes and do so with less bias. These models are already informing mental health resource allocation and tailored treatment planning in fields like oncology and cardiology.
Ultimately, the future of medical AI hinges not just on what it can do, but how—and for whom—it is built.
A New Medical Era
From protein folding to diagnostics, AI is driving a revolution in medicine and science. What distinguishes 2025 from earlier years isn’t just performance—it's integration. AI is no longer just assisting scientists and doctors; it's collaborating with them, shaping discoveries, optimizing workflows, and guiding decisions.
Still, challenges remain: datasets must become more inclusive, workflows must adapt to new tools, and ethical concerns must be proactively addressed. But with thoughtful deployment and continued research, the future of AI in medicine looks not just promising, but transformative.
References
[1] Maslej, N., Fattorini, L., Perrault, R., Gil, Y., et al. (2025). The AI Index Report 2025. Stanford University, Human-Centered AI (HAI).
[5] Lutsker, G., Sapir, G., Shilo, S., Merino, J., Godneva, A., Greenfield, J. R., ... & Segal, E. (2024). From glucose patterns to health outcomes: A generalizable foundation model for continuous glucose monitor data analysis. arXiv preprint arXiv:2408.11876.
[6] Measuring Intelligence: Key Benchmarks and Metrics for LLMs, Transcendent AI
[7] Advancing medical AI with Med-Gemini, Google
[8] Chen, Z., Varma, M., Delbrouck, J. B., Paschali, M., Blankemeier, L., Van Veen, D., ... & Langlotz, C. (2024). Chexagent: Towards a foundation model for chest x-ray interpretation. arXiv preprint arXiv:2401.12208.
[9] Guevara, M., Chen, S., Thomas, S., Chaunzwa, T. L., Franco, I., Kann, B. H., ... & Bitterman, D. S. (2024). Large language models to identify social determinants of health in electronic health records. NPJ digital medicine, 7(1), 6.
Comments